Ever stared at a spreadsheet full of campaign data and felt completely lost? You’re not alone. Many marketers feel overwhelmed when it comes to analysing numbers. But here’s the good news—you don’t need a maths degree to make smart, data-driven decisions.
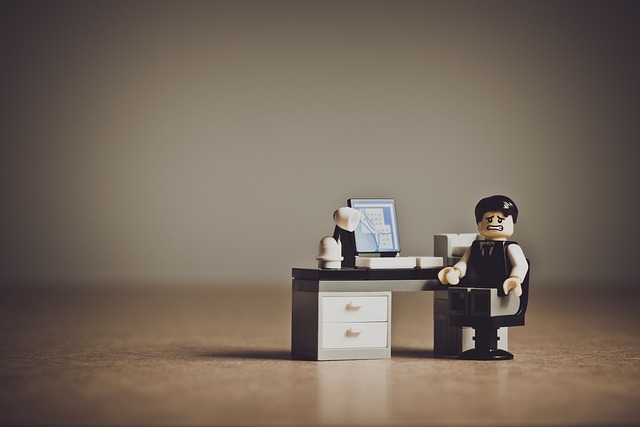
Let’s walk through the statistics concepts that will actually help you in your marketing work, explained in plain English.
The basics you’ll use every day
Averages: More than just a simple number
When you look at your marketing performance, averages quickly become your friend. But which average should you use?
- Mean: The one we typically call “the average”—add all values and divide by how many there are. Great for metrics like average order value.
- Median: The middle value when arranged in order. Perfect for understanding “typical” customer behaviour without being skewed by outliers.
- Mode: The most common value in your dataset. Useful for identifying your most popular products or most common customer behaviours.
Why does this matter? Imagine your campaign generated sales ranging from £20 to £5,000. The mean might be £300, but if most people spent around £50 (the median), that tells a different story about your typical customer.
Percentages and percentage change
Simple yet powerful. Percentage change helps you understand growth or decline in key metrics:
Percentage change = ((New value – Old value) / Old value) × 100
Remember that a 50% decrease followed by a 50% increase doesn’t get you back to where you started. This matters when analysing recovery after marketing budget cuts.
Distribution: How your data spreads out
Understanding distribution helps you see the full picture beyond averages.
- Normal distribution: The famous bell curve where most values cluster around the middle. Many natural phenomena follow this pattern.
- Skewed distribution: When values bunch up on one side with a “tail” on the other. Often seen in income data or social media engagement metrics.
Knowing your distribution shape helps you choose the right analysis methods and avoid misleading conclusions.
Moving beyond the basics
Statistical significance: Is that result real or random?
Ever run an A/B test and wonder if the winner really won, or if it was just luck? Statistical significance helps answer this question.
The concept boils down to: “How likely is it that the difference we’re seeing happened by pure chance?”
The most common measure is the p-value:
- A p-value of 0.05 means there’s a 5% chance your result occurred randomly
- The lower the p-value, the more confident you can be that your result is “real”
For marketing, this matters when deciding if that new email subject line actually performed better or if the improvement was just random variation.
Correlation: When things move together
Correlation measures how strongly two variables are related, on a scale from -1 to +1:
- +1: Perfect positive correlation (as one increases, so does the other)
- 0: No correlation
- -1: Perfect negative correlation (as one increases, the other decreases)
You might discover that email open rates strongly correlate with time of day, or that social media engagement weakly correlates with sales.
But remember the golden rule: correlation doesn’t equal causation. Just because two things move together doesn’t mean one causes the other.
Regression analysis: Finding relationships in your data
Regression helps you understand how one variable affects another. It’s like correlation but more powerful.
For example, linear regression might help you understand:
- How much an additional £1,000 in ad spend increases sales
- The impact of email frequency on unsubscribe rates
- How product pricing affects purchase volume
The basic form looks like:
Y = a + bX
Where Y is what you’re trying to predict, X is your input variable, and a and b are constants.
Practical applications in marketing
Segmentation and cluster analysis
Grouping similar customers together helps you target them more effectively. Cluster analysis finds natural groupings in your data based on multiple variables.
This might reveal that your customers fall into three distinct groups with different buying patterns—information you can use to personalise marketing messages.
Predictive modelling: What will happen next?
Beyond understanding what happened, statistics helps you predict what will happen:
- Forecasting: Projecting future sales based on historical data and trends
- Propensity modelling: Calculating the likelihood that someone will convert, churn, or respond to a specific offer
- Attribution modelling: Determining which marketing touchpoints deserve credit for conversions
These techniques help you allocate your budget more effectively and focus on high-potential customers.
Confidence intervals: Managing uncertainty
Rather than a single number, confidence intervals give you a range where the true value likely falls. For example:
“Our conversion rate is 3.2%, with a 95% confidence interval of 2.9% to 3.5%.”
This acknowledges the uncertainty in your data and helps you avoid overreacting to small fluctuations that might just be random noise.
Making statistics work for you
Statistics becomes truly valuable when you use it to answer specific business questions:
- “Which customer segments are most profitable?”
- “Which marketing channels deliver the best ROI?”
- “What’s the optimal frequency for our email campaigns?”
- “How many samples do we need for a reliable survey?”
With the right statistical approach, these questions become answerable, and your marketing becomes more effective.
Leave a Reply